Defining the functional and anatomical circuitry of Parkinson's disease motor symptoms
Toward symptom-specific closed-loop deep brain stimulation
Parkinson’s disease (PD) is a complex neurodegenerative disease with heterogeneous motor phenotypes. In order to design symptom-specific closed-loop deep brain stimulation (DBS), we simultaneously quantified several metrics of PD motor dysfunction (e.g. tremor, slowness) using a target-tracking task.
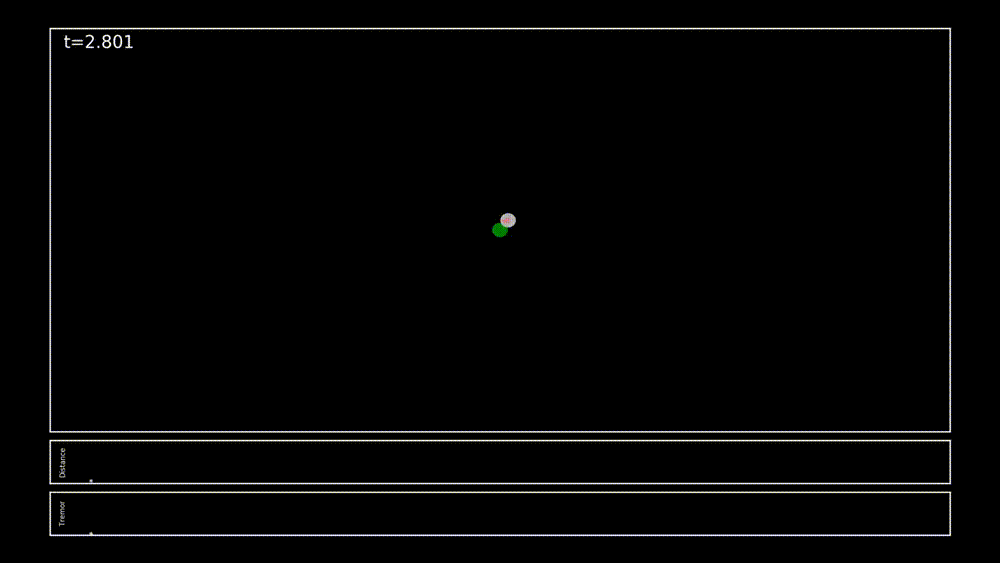
When administered in the clinic, we were able to use simple machine learning algorithms (support vector machines) to differentiate the behavior of patients with movements disorders (PD, essential tremor - ET) from age-matched controls. In addition, we were able to characterize the unique behavioral phenotype of PD from ET.
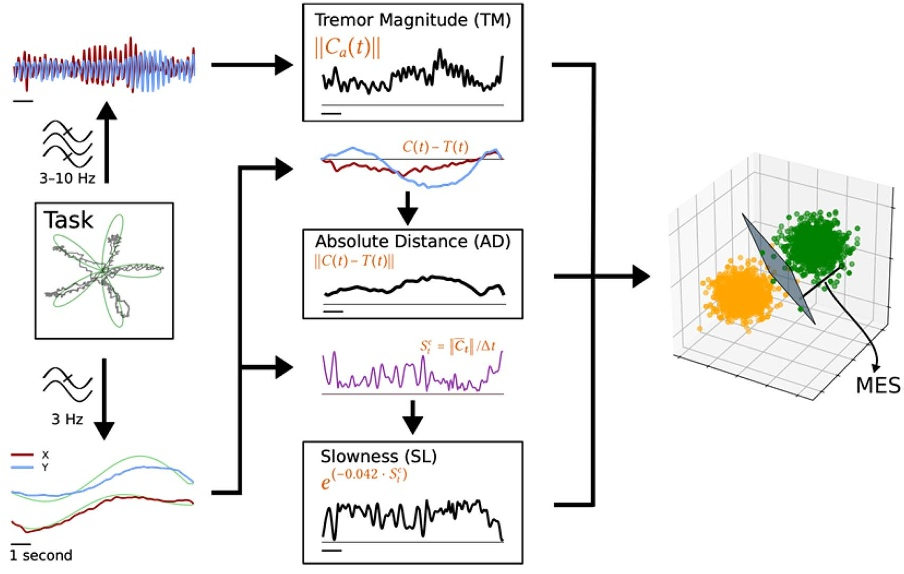
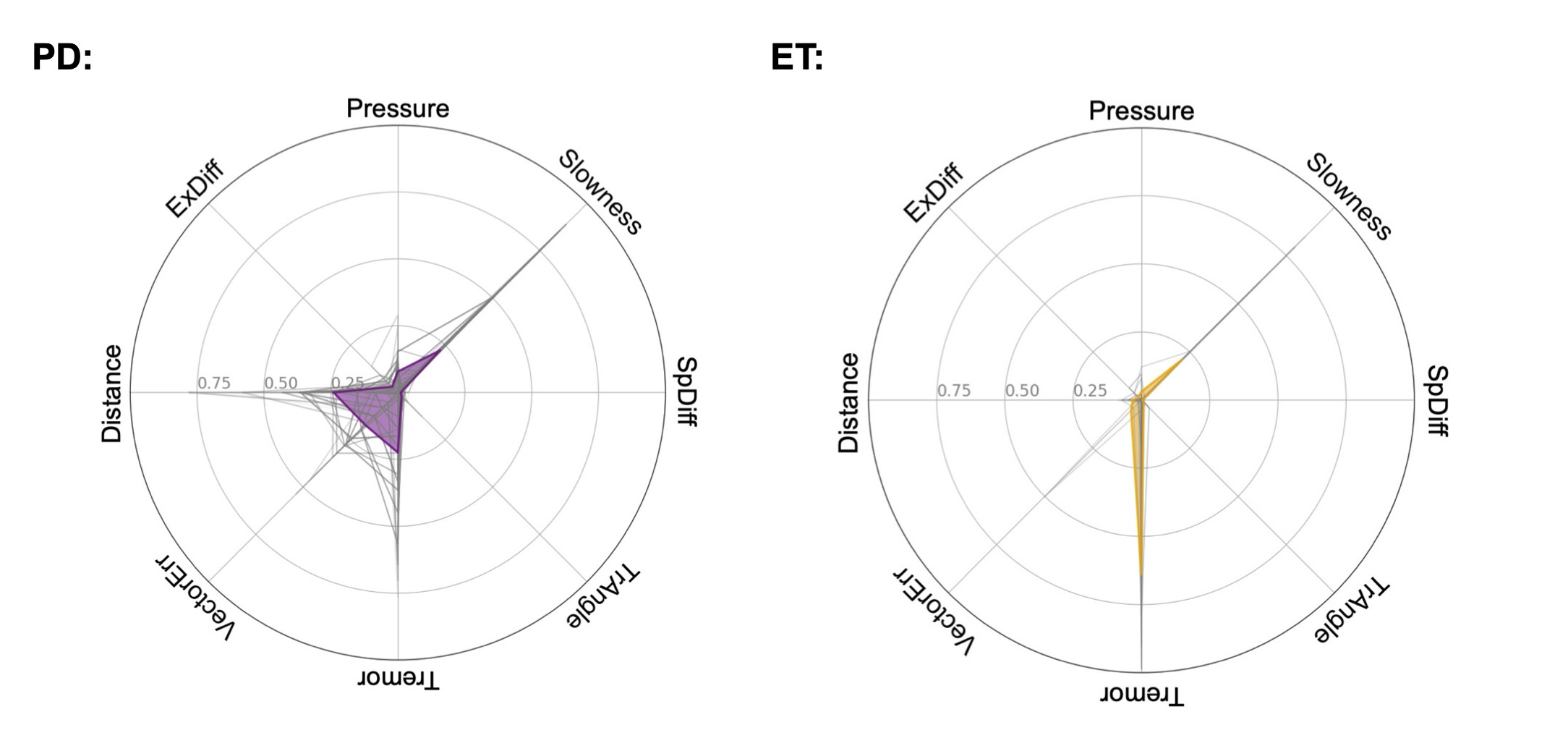
We then had patients with PD perform our task to the operating room as they underwent awake implantation of DBS electrodes. Using microelectrode recordings from the subthalamic nucleus (STN), we were able to decode the presence of PD motor dysfunction on short-timescales (i.e. seconds). Similarly to how PD has diverse phenotypes, optimal neural decoding models were patient-specific and relied on a variety of frequency bands throughout the local field potential (LFP) spectrum.
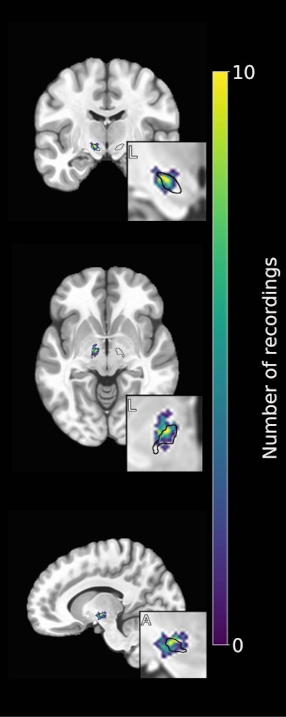
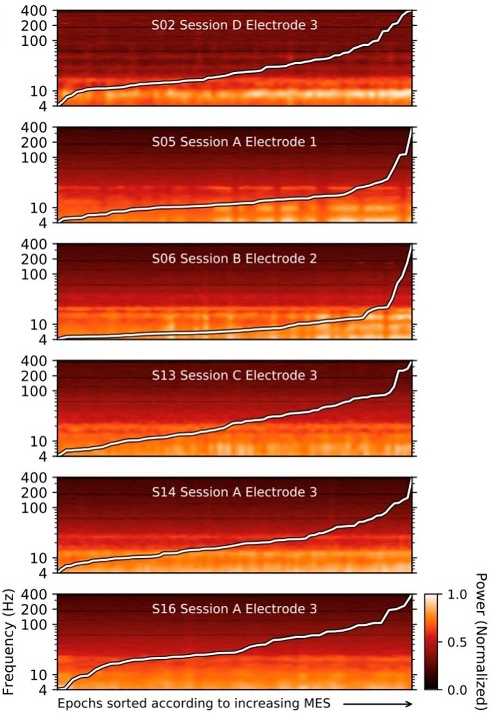
Although our method of patient-specific symptom decoding was flexible to each patient’s constellation of symptoms, our task also provided the opportunity to define the neurophysiologic representations of individual motor symptoms such as tremor. By combining STN microelectrode and electrocorticography (ECoG) recordings, we were able to elucidate that tremor emerges due to tremor-frequency (3-8 Hz) oscillations in the STN, but is sustained by cortico-cortical oscillations at higher frequencies (12-20 Hz).
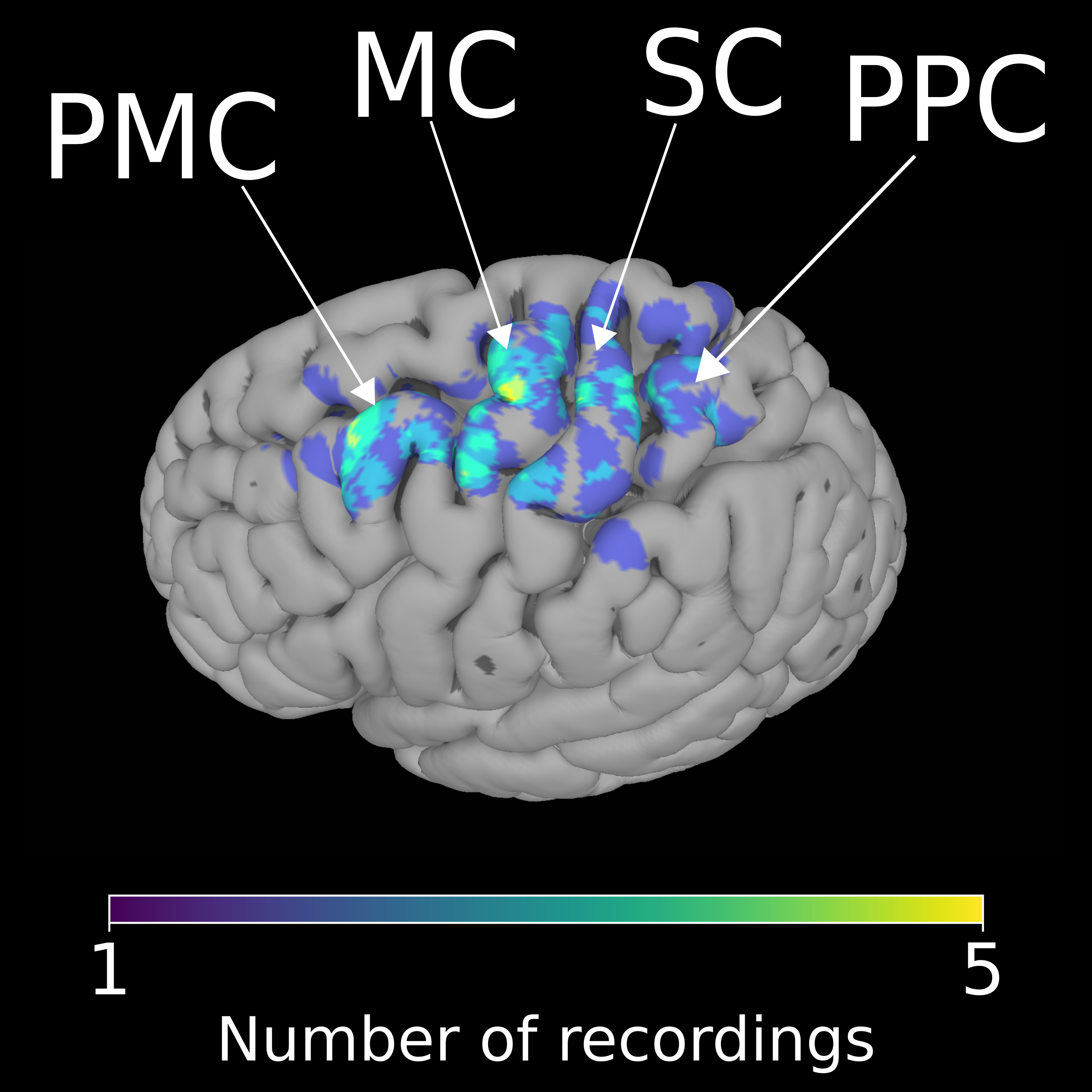
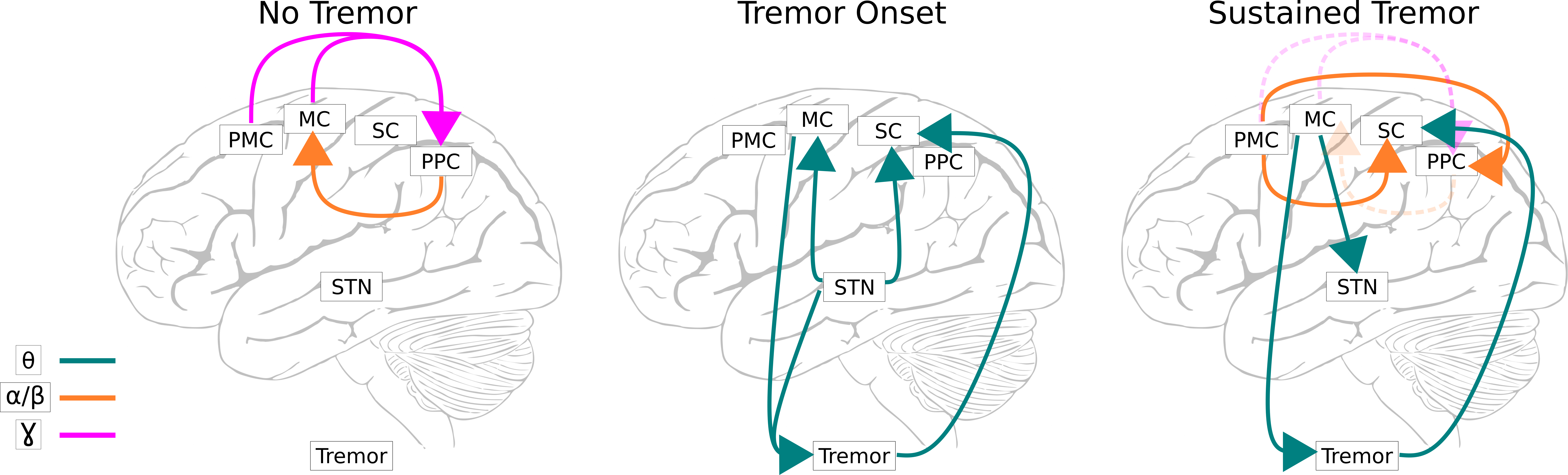
We then investigated how these different motor phenotypes can individually be represented and decoded by our machine-learning approach. Not only were we able to identify distinct spectral signatures of individual Parkinsonian motor features, but we also demonstrated that optimal tremor and bradykinesia feature decoding arose from distinct subregions of the STN.
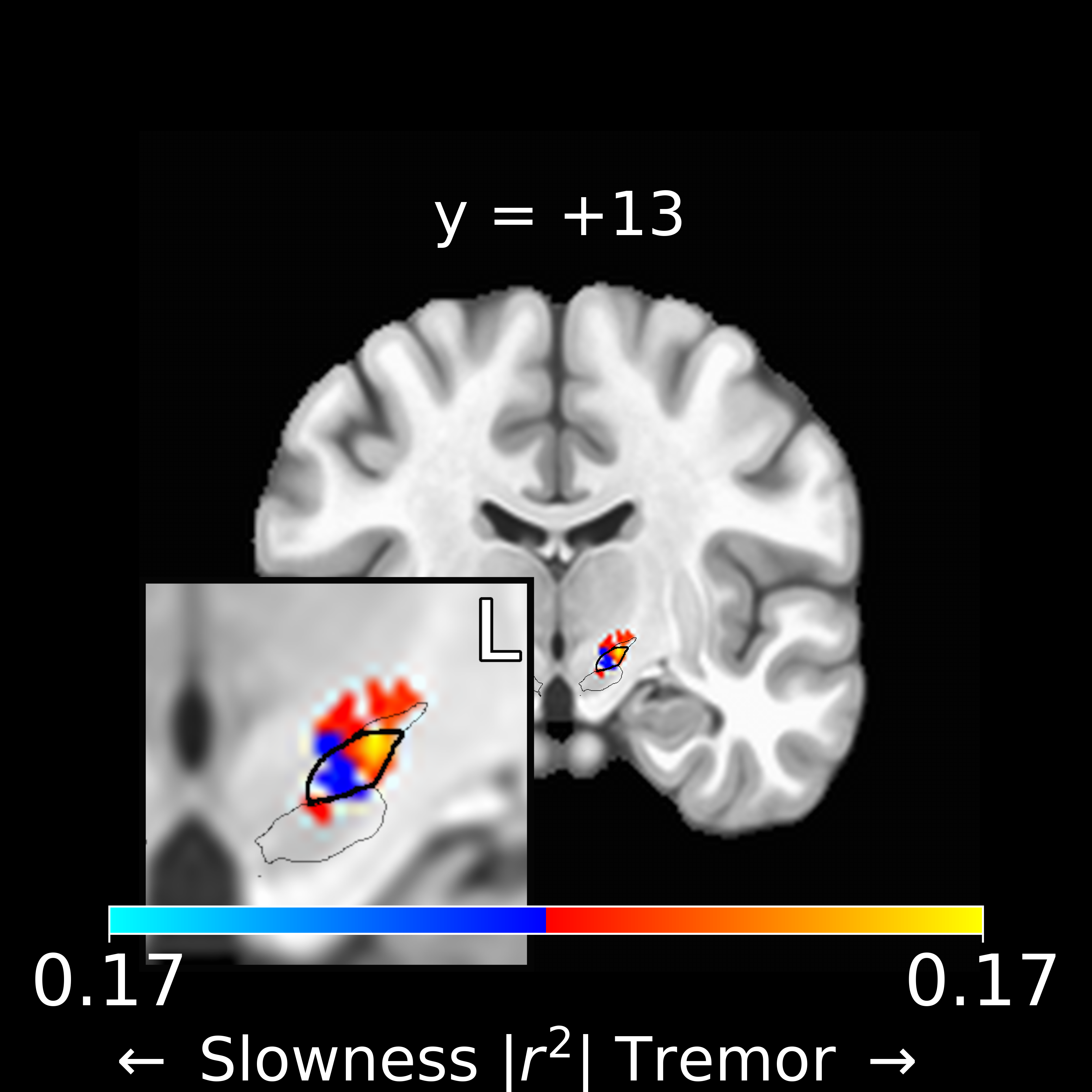
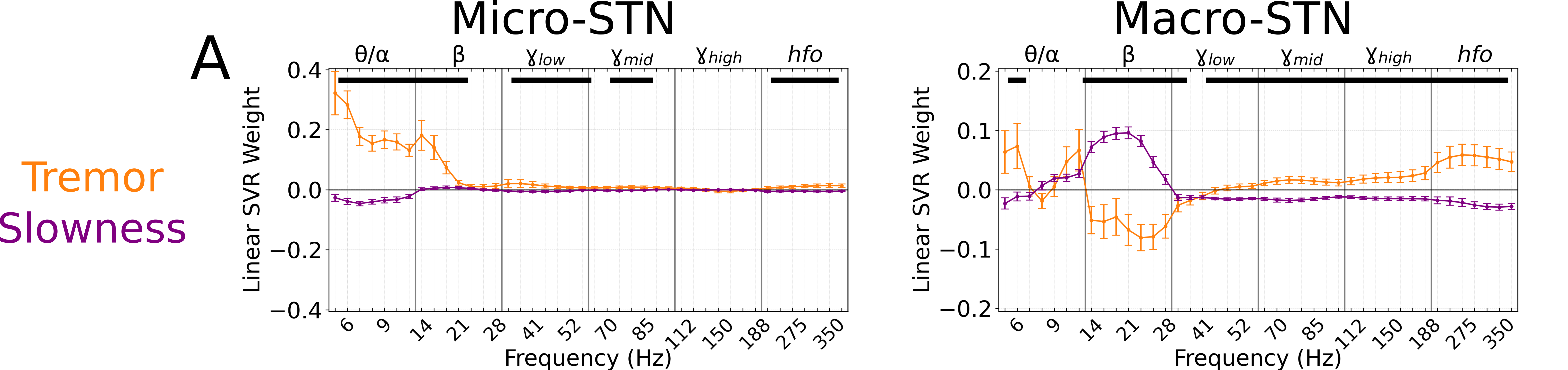
Future efforts are applying this knowledge to inform adaptive closed-loop deep brain stimulation protocols.